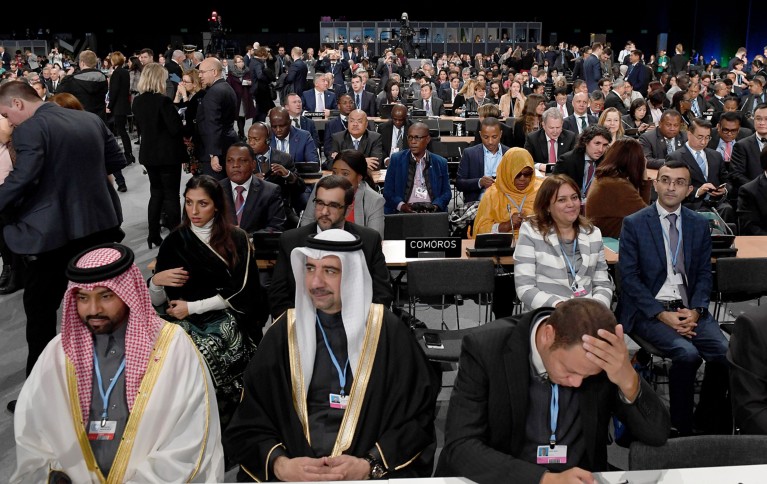
Speeches from international diplomatic sessions were used to train a machine-learning translation system.Credit: Janek Skarzynski/AFP/Getty
The dream of a Babel fish — the translating animal envisioned by classic science-fiction franchise The Hitchhiker’s Guide to the Galaxy — could be a bit closer to reality. Researchers at tech giant Meta have created a machine-learning system that almost instantaneously translates speech in 101 languages into words spoken by a voice synthesizer in any of 36 target languages.
The Massively Multilingual and Multimodal Machine Translation (SEAMLESSM4T) system can also translate speech to text, text to speech and text to text. The results are described in Nature on 15 January1.
Meta, which is headquartered in Menlo Park, California, and runs social-media sites such as Facebook, WhatsApp and Instagram, says it is making SEAMLESSM4T available open-source for other researchers who want to build on it, following the success of releasing its LLaMA large language model to developers worldwide.
Data scarcity
Machine translation has made huge strides in the past few decades, thanks in large part to the introduction of neural networks that are trained on large data sets. Training data abound for major languages — especially English — but are notoriously scarce for many other languages. That inequality has limited the range of languages that machines can be trained to translate. “This affects any languages that appear infrequently on the Internet,” writes Allison Koenecke, a computer scientist at Cornell University in Ithaca, New York, in a News & Views article that accompanies the paper.
Robo-writers: the rise and risks of language-generating AI
The team at Meta built on its previous work on speech-to-speech translation2 as well as on a project called No Language Left Behind3, which aimed to provide text-to-text translation for some 200 languages. Through experience, researchers at Meta and elsewhere have found that making translation systems multilingual can improve their performance even in translating languages with limited training data; why this happens is unclear.
The team collected millions of hours of audio files of speech, along with human-generated translations of that speech, from the Internet and other sources, such as United Nations archives. The authors also collected transcripts of some of those speeches.
The team also used reliable data to train the model to identify two matching pieces of content. This allowed the researchers to pair around half a million hours of audio with text and automatically match each snippet of one language with its counterpart in others.