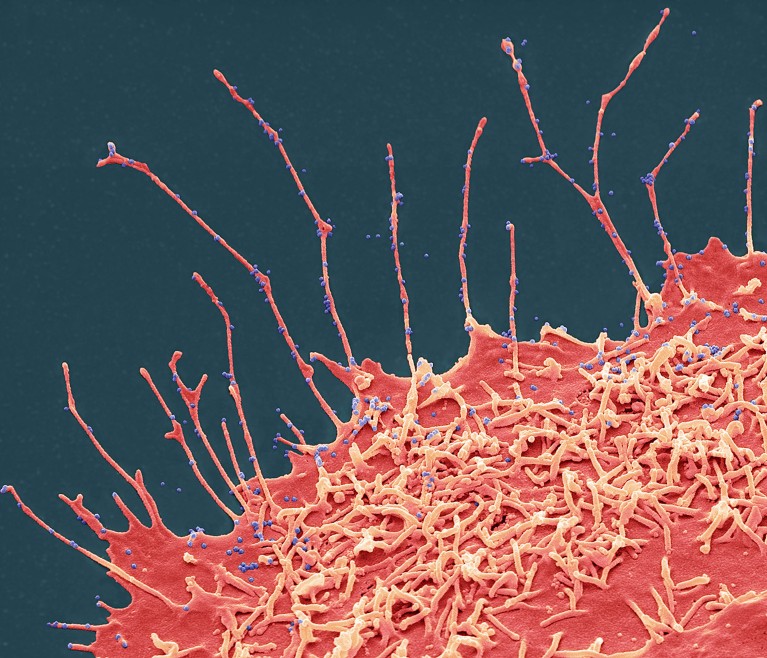
RNA viruses including SARS-CoV-2 constantly accrue new mutations.Credit: Steve Gschmeissner/Science Photo Library
The holy grail of pandemic preparedness is being able to predict how a virus will evolve just by looking at its genetic sequence. Those days are still a way off, but a growing number of research groups are using artificial intelligence (AI) to predict the evolution of SARS-CoV-2, influenza and other viruses.
Viruses — particularly RNA viruses such as SARS-CoV-2 — constantly evolve by accruing new mutations. Some of these changes are advantageous to the virus, allowing variants to evade host immunity and spread rapidly. By predicting how a virus will evolve, researchers could, in theory, design vaccines and antiviral treatments ahead of time.
So far, AI tools can predict which single mutations in a virus will be most successful, and which variants will ‘win’ out in the short term. But they are still far from being able to forecast combinations of mutations or variants that will occur a long time in the future.
It’s “a really exciting and very useful area for research”, says Brian Hie, a computational biologist at Stanford University in California, who was among the first researchers to apply large language models to the study of viral mutations1. But predicting viral evolution is still extremely challenging, he says.
AI tools
In the past, researchers have conducted laboratory experiments to identify variants with enhanced properties, but these are laborious and time-consuming. Some groups, such as the lab led by Yunlong Cao, an immunologist at Peking University in Beijing, have developed experiments investigating how individual mutations affect a virus’s ability to escape detection by a panel of antibodies2. These experiments can explain a large amount of viral evolution, but not all of it.
The arrival of AI-based protein-structure prediction tools — such as AlphaFold, created by London-based AI company DeepMind, as well as ESM-2 and ESMFold, both created by Meta (formerly Facebook, headquartered in Menlo Park, California) — has brought new energy to the field, says David Robertson, a virologist at the University of Glasgow, UK.
AI models require vast amounts of data to be able to predict viral evolution. The mass sequencing of SARS-CoV-2, the virus that causes COVID-19, has made this possible, says Jumpei Ito, a bioinformatician at the University of Tokyo. Researchers now have close to 17 million sequences they can use to train their models.
One model, called EVEscape, which was developed by Debora Marks at Harvard Medical School in Boston, Massachusetts, and her team, has been used to engineer 83 possible versions of the SARS-CoV-2 spike protein, which the virus uses to infect cells. These spike avatars can evade antibodies produced by people who have been vaccinated or infected with currently circulating variants4, and could be used to test the effectiveness of future COVID-19 vaccines.
Ito’s group is focusing on a broader characteristic of viral fitness — the ability of variants to spread rapidly in a population, and eventually dominate. The researchers used ESM-2 to create a model called CoVFit, which can predict the relative fitness of SARS-CoV-2 variants. CoVFit is trained on 13,643 SARS-CoV-2 spike-protein variants, and also uses experimental data from Cao’s group about how individual mutations affect the virus’s ability to evade antibodies. Ito’s team created a restricted model trained using variant data up to August 2022, and found that it successfully predicted the improved fitness of certain variants after that cut-off — including XBB5, a novel variant that took hold later that year.
By March 2024, the dominant SARS-CoV-2 variant worldwide was one named JN.1. Using CoVFit, Ito’s group identified three single-amino-acid changes that would help JN.1 to gain fitness. These mutations have since been seen in variants that are rapidly expanding globally.