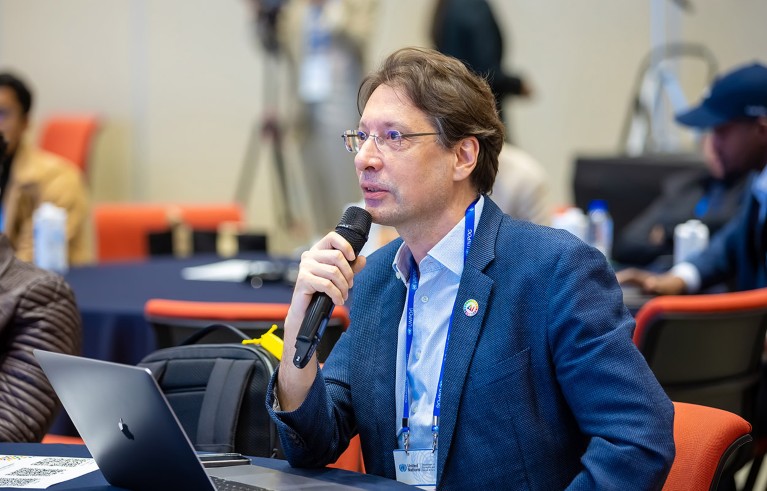
Serge Stinckwich is working to understand how to use AI tools sustainably.Credit: UNDESA/DPIDG/UNPOG
There is a lot of interest from inside the United Nations around how artificial intelligence (AI) can be used to speed up progress towards its 17 Sustainable Development Goals (SDGs), says computer scientist Serge Stinckwich.
As head of research at the United Nations University Institute in Macau (UNU Macau), which was established by the UN in 1992 to do research and training on the use of digital technologies in addressing global issues, Stinckwich is interested in how AI can help countries to hit their SDG targets by the 2030 deadline.
Any gains made using AI will come with costs, however. A notoriously power-hungry resource that is vulnerable to bias and inequitable access, AI presents its own challenges.
Stinckwich spoke to Nature Index about how institutions can use AI tools responsibly to power their SDG-related research.
What is one example of how AI can be used to speed up progress towards the SDGs?
The popularity of large language models (LLMs) has caused a rapid escalation in the amount of data being used to train AI systems. There’s now a scarcity of machine-readable, diverse data on the Internet for training AI algorithms. Synthetic data, which are generated using algorithms and simulations that mimic real-world scenarios, provide a way to train AI models on more data than would usually be possible.
Synthetic data can help to rebalance biased data sets — for example, in a data set skewed towards one gender, synthetic data can be added to balance representation. They can also help to address the problem of scarcity or missing data. This can be particularly useful in medical research, in which people’s health data and personal information can be hard to obtain because of privacy issues.
This approach will become increasingly common. Gartner, a research and consulting firm headquartered in Stamford, Connecticut, predicts that by the end this year, more than 60% of the data used to train machine learning models will be synthetic.
What are the risks in using synthetic data?
Synthetic data are generated from data sets that already exist. So, biases in the initial data sets could be propagated throughout the synthetic data, and in turn, AI models that have been trained on them. Our work at UNU Macau focuses on understanding the impact of synthetic data used in machine learning, including the risks for sustainable development through research.
Last year, for instance, we published a technology brief in which we tried to identify the benefits and risks of using synthetic data in AI training. On the basis of this work, we proposed guidelines for responsible use of synthetic data in research related to SDGs, especially in poorer countries. This includes using diverse data when creating synthetic data sets, which means including a wide range of demographics, environments and conditions. We also recommend disclosing or watermarking all synthetic data and their sources, disclosing quality metrics for synthetic data and prioritizing the use of non-synthetic data when possible.
2024 Research Leaders
We also recommend that institutions and organizations establish global quality standards, security measures and ethical guidelines for generation and use of synthetic data.
We hope that UN member states and agencies will adopt our guidelines to support policy-making in the global governance of AI.
What other AI tools or resources are making a difference in SDG-related research?
When I was a researcher at the French Research Institute on Sustainable Development (IRD) in Marseille, I worked on a project called Deep2PDE in Cameroon. Together with colleagues at the local universities, our team used machine-learning tools to understand how competition for light between plant species affects agroforests in which cocoa trees are grown alongside other trees and crops. This helped us to simulate, design and test systems to optimize cocoa production.
There are lots of practical applications of AI, such as this one, that can aid progress towards the SDGs. A big advantage is that these tools can help teams to tailor their work to the needs and contexts of communities; what might be useful for people in Europe or North America might not work in Africa.
What are the other risks of using AI more generally to progress SDG research?
We need big computing infrastructure to power AI systems, and this requires resources such as water for cooling systems. This has implications for sustainability, and by extension, the SDGs. So, we have to be cautious. The environmental impacts of AI systems, including on the use of minerals and water and greenhouse-gas emissions, is a big concern. For instance, some research suggests that training an LLM, such as the one powering the chatbot ChatGPT, could produce carbon emissions equivalent to those from roughly 500–600 flights between New York City and Los Angeles, California.
Some technology companies are not keen to share the actual cost of their AI systems and the resources they use. This makes it difficult for researchers to evaluate the environmental impacts of AI and to advise governments and policymakers on how to mitigate them.
Another major issue is one of inequity: AI tools and data are often owned and controlled by companies and institutions in richer countries, so poorer countries are limited in how they can use them to further their SDG-related research.
How can the equity problem be addressed?
A big reason for this issue is that most of the progress in building LLMs in the past few years has been done by private companies, not by academics and research institutions. Some potential solutions include creating public–private partnerships and initiatives to democratize access to computing infrastructure.
For example, the Swiss International Computation and AI Network, run by the Swiss Federal Institute of Technology in Zurich, aims to give researchers from low-income settings access to supercomputing resources so that they can develop AI tools that benefit the world. They’re partnering with organizations such as Data Science Africa, a non-profit group in Nairobi, to empower young Africans to use data science to develop solutions for local problems and to help reduce inequalities in data and software infrastructures.
Some online platforms, such as the one run by Hugging Face, a technology company in New York City, make AI-tool-building infrastructure accessible to everyone. It’s open-source, allowing users to share and access resources, including data sets and models developed by others. This approach can help to reduce resource consumption and the environmental impact of AI development.
This interview has been edited for length and clarity.
Nature Index’s news and supplement content is editorially independent of its publisher, Springer Nature. For more information about Nature Index, see the homepage.